Use of Artificial Intelligence in the 100% Visual Inspection of Parenterals
Artificial intelligence or rather machine learning has the potential to change patient care in the healthcare industry by gaining new insights based on the extensive amounts of data generated during the manufacture and production of pharmaceutical products. The pharmaceutical industry's interest in using artificial intelligence - especially in the visual control of parenterals - is increasing considerably due to the high expectations on the harnessing of potential for optimisation so far unused.
The 100% visual inspection of injectables serves to ensure the product quality within the production process and is regulated by several pharmacopoeias and guidelines (USP, Ph. Eur., EU-GMP Annex 1, etc.).
So far, there are no specific regulatory requirements for the validation of artificial intelligence used in the visual inspection of parenterals. From the GMP point of view this may be considered as being problematic. In particular, the importance of the data available for training and validation, the model design based on it, and the question how to ensure the quality and integrity of the data throughout the entire product life cycle are unclear and often underestimated. Discussions about appropriate validation concepts by technical committees, communities and regulators have begun, but are still at a very early stage and often remain theoretical, based on nice concept slides. Many users and other stakeholders remain uncertain about how to deal with the new technology.
The present article refers to the support of automated inspection machines (AIM) by machine learning models aiming to improve the efficiency or rather detection performance of these machines. Furthermore, questions about the establishment of artificial intelligence (AI) in the regulated industry, technological aspects and risk-based validation approaches are discussed. In addition, the article aims to provide some criteria to assist in identifying possible areas of improvement, taking into account costs and benefits
Inadequately adapted equipment
Despite sophisticated inspection concepts, such as high-resolution cameras and optics, selected illuminations, customized feeding mechanics and presentation of the objects, fully automated visual inspection machines operate at technical limits. Due to the variation in process and packaging material in addition to multiple dosage forms to be supported, it is often not possible to maximise economic interests and minimise compliance risks at the same time.
Since the core competence of the supplier typically lies in the construction of machines and less in the knowledge of the pharmaceutical daily routine operation and at the same time the customer cannot provide the necessary technical skills for automation and plant construction, fully automated inspection machines are often specified insufficiently already in the course of procurement projects. Consequently, the inadequately adapted inspection machine can only be operated under real conditions at the expense of large losses (due to false rejections). For the customer, this is the start of an optimisation process that can last for years.
New Possibilities given by the use of AI
Artificial neural networks are algorithms that are modelled based on the human brain. A sufficiently adapted model makes it possible to solve complex tasks in the fields of statistics, computer science and economics with the help of a computer. Data sources such as images, sounds, documents, charts or time series can be interpreted and information or patterns extracted. Applied to new process data, this allows predictions for the future to be made. Based on today's knowledge, automated visual inspection (AVI) can also benefit from the use of artificial intelligence by making inspection recipes available for commercial use more quickly while improving the distinction between conforming and non-conforming units.
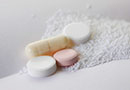
Recommendation
14-16 October 2025
Granulation & Tableting - Live Online Training
Creation of a machine learning model
In visual inspection, the human operator is still considered the "gold standard" delivering consistently reliable and robust results - at least over limited periods of time and volumes. In order to replicate human decision-making structures, artificial neural networks are being developed whose complexity and topology must be "adapted" depending on the inspection task.
Artificial neural networks are divided into layers. During the design phase, the aim is to identify the neural network in a given family of neural networks that is well adapted to the task's requirements and can be used efficiently and in a resource-saving manner. Once the architecture has been defined, the training phase follows, in which the network is trained with input-output-data-pairs.
In our example, a data pair consists of the image of a product (input) and the expected result (output). Based on the current standard for supervised learning in the regulated industry, the result is assigned to an image through human interaction as part of the labelling process. The quality of the data set controlled by humans (the so-called 'human-in-the-loop' - HITL) ensures that the network makes the right decisions.
By training and validation of the network, a function is generated that ideally recognises new variants or rather images of the product correctly, assigning the expected result. The abstraction of such a network is often referred to as a 'model'.
The model is well adapted and can be used in regulated environments if it can predict and generalize robustly and repeatably beyond the characteristics represented in the training data.
Challenges
Until now, recipes for inspection machines have been created by vision technicans. The creation and monitoring of proper function is based on the training and qualification sets available. The suitability and performance of the machine in everyday use depends largely on the competence and expertise of the vision expert in adapting the recipe. This is followed by the optimization phase. Typically, this is a continuous process that must be planned and monitored as part of life cycle management. If you believe the discussions about the seemingly unlimited field of possible applications, one might think that artificial intelligence will replace experts in the future, solving problems of this kind without human interaction. In reality, however, this does not work any more than purchasing an inspection machine without expert knowledge.
Fig. 1: Challenges
A crack in the camera's image is often difficult to distinguish from an artefact, i.e. a structure caused by the optics (reflection, blurring, etc.), please refer to the 'Challenges' image. Particularly in the case of boundary samples, i.e. units that cannot be distinguished by means of unique, 'strong' features, it is difficult or even impossible to classify the product correctly. However, since the normal user is typically not an expert in image processing and the data scientist usually has no experience in process and product knowledge, these challenges can only be met satisfactorily if it is possible to mediate between both parties and establish a mutual understanding.
In the worst case, neither the user nor the data scientist knows that the algorithm was developed on incomplete, non-representative data and thus compliance risks may remain undetected. This applies to the abstraction of models for rule-based, traditional image processing algorithms, as well as in the context of machine learning, where the data scientist groups the available images into data sets in order to train the model. For illustration and better understanding, see pictures of the creation and subsequent performance of a model (steps 1-4).
Comparable to the human learning process, also the model must be trained and validated with specially created and balanced data sets, whereby the effort largely depends on the complexity on the one hand and the error costs of the application on the other.
Fig. 2-5: Steps 1-4
Risks of a machine learning model
The potential risks of a machine learning model basically depends on the area of application and the associated costs of a wrong decision. For visual inspection, one should take into account the criticality of the manufacturing process in which the model is involved, the inherent patient's risk, e.g., due to potential sterility problems, and additional controls in place to mitigate risks, such as sampling schemes indexed by acceptance quality limits (AQL) for lot-by-lot inspection.
Neural networks identify patterns in training data, using them to predict future data. Similar to humans, who, emotionally influenced, tend to interpret information in a way confirming previous expectations ("confirmation bias"), the same applies to machine learning.
Unbalanced, unspecific training data, for example, can lead to irrelevant structures being focused ("overfitting"), or the model being oriented towards dominant features ("biasing"). If, on the other hand, relevant characteristics are not sufficiently represented in the training data, this is referred to as "underfitting". In both cases, the assumptions derived from the data do not allow reliable predictions under realistic conditions.
While the risks mentioned above must be considered when developing and training models, companies are realizing that maintaining the model in the lifecycle plays an equally, if not more, important role.
For example, incomplete documentation and management of models used in production and the lack of knowledge which data was used to create a specific model can lead to data integrity risks: e.g., models, created at an early stage, do not meet current organizational requirements for testing and documentation, dependencies between models in production are not recognized, or inadequate monitoring of models in the life cycle leads to a deterioration in performance, to name just a few.
Risk mitigation
Technical risks arising from design, training and validation have a direct impact on the safety and performance of the model. These risks can be avoided or rather be controlled if comprehensive domain knowledge can be made available throughout the entire lifecycle. To promote appropriate performance of the model for the target application, to avoid any biases or errors and to predict circumstances under which the model might underperform (e.g. data set drift), characteristics and variations of the product and manufacturing process must be sufficiently represented in the training and validation data sets.
Since training and qualification kits available are usually artificially produced, their number is limited and thus their representativeness is somewhat restricted compared to the reality of production, data sets used for training and validation must be enriched with data from production as well as with artificially created data. The composition and quality of such "reference standards" is of particular importance from a GMP perspective and, in addition to the creation, qualification and maintenance of the neural networks in the life cycle, should be regulated based on a risk-assessment and documented in appropriate SOPs. A model management strategy following the ALCOA principles can help to describe the scope and use of the models, to regularly track them and thus to ensure traceability.
Establishment and Qualification of a Model in the Life Cycle
The model‘s life cycle can be divided into five phases:
Identification of possible areas of improvement
The first step in the life cycle of a model is to identify, analyze and describe the need for optimization ("Identify"). In principle, the events that trigger the creation or modification of a model can be divided into planned and unplanned events:
Introduction or optimization of models as part of planned events:
Changes triggered by the purchase of a new inspection machine, accompanying product transfers or by the establishment of new control processes, as well as changes proposed to increase the efficiency, function or robustness of existing processes.
Changes due to unplanned quality and compliance events:
Changes that are necessary due to a quality or regulatory compliance events (e.g., deviations, CAPAs or authority requirements). These include, for example, systematically missed defects that were sorted out by the machine as conforming products, e.g. by AQL testing
Requirements specification
In the specification phase, the problem and requirements are captured and documented. These include product, dosage form and packaging specifications, machine properties (type, camera stations, speed, etc.), product quality requirements (e.g., defects and their prioritization according to criticality) and challenges in classifying defects, such as ambiguities due to the inability distinguishing between microbubbles, particles or other artifacts, i.e., borderline cases.
The required data sets are based on artificially created samples, such as the qualification kits. In addition, real data from production is required, which, enriched by artificial created data, should close the "reality gap".
Once the inspection problem is understood and the initial data is available, a labeling guidance must be agreed between the user and supplier, communicated and made available to the labeling team members.
Creation, training and validation of the model
Based on the data sets provided and labeled, the model is designed, trained and validated. In order to control possible effects on the safety and performance of the model, the model building risks known from traditional image processing must be taken into account (see section "Risks of a machine learning model ").
Training and validation data sets must be selected and managed in ensuring that they are independent of each other. Relevant information for the unique identification and differentiation of data sets must be recorded and documented, e.g. machine, product, camera station, labeling or numbering of the sample, expected result, etc.
Qualification strategy
After confirming the expected function during validation, the model is "frozen" and the detection performance is confirmed using the qualification kits. This step should be carried out following the traditional validation for comparability and explainability, even if the artificially created qualification kits are not necessarily meaningful for confirming the performance.
However, it is at least equally important to ensure that the risk-based specifications for creating the model have been adhered to and thus to comply with the recognized "Good Machine Learning Practices (GMLP)", please refer to section "Risk mitigation". As already mentioned several times before, particular attention must be paid to the composition and representativeness of the training and validation data.
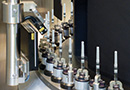
Recommendation
Vienna, Austria21-23 October 2025
Visual Inspection of Parenterals PLUS Pre-Course Fundamentals of Visual Inspection
Operation
After qualifying, it is advisable to verify the correct functioning of the model as part of regular production. Demonstrating the expected behaviour as part of process validation promotes trust and also enables new insights. Comparing the traditional recipe with the decision of the machine learning model as part of benchmarking can also help to promote trust in the new technology.
Previously defined measures for the continuous monitoring and maintenance of models as well as for expanding the data-driven process understanding should be followed up along the life cycle in order to mitigate the risks mentioned above.
Conclusion
Assuming that visual inspection contributes significantly to the manufacturing's risks, typically up to 30.000 labeled images per camera station must be provided to create a model ensuring robust detection performance, even across the training data.
Even if the integration and qualification of AI-supported systems initially involves a lot of effort and the risks are not yet widely understood, the structured collection and review of data leads to "cumulative process and product knowledge" that can be transferred to other products and applications. By using technologies such as transfer learning, the development and commissioning phase for new recipes and processes that is common today can be significantly shortened and, in addition, standardized in terms of quality.
But be careful! Even if freely available tools such as the interactive, web-based computing platform Jupyter Notebooks seem to promise successful results on selected training data, you may already be in the 'overfitting' trap without realising it. Ultimately, data science is not something that can be learnt and understood in depth in a weekend with just a few clicks.
Anyone who has high demands on product quality (lowering "false acceptance rate") and at the same time on the efficiency (lowering "false reject rate") of an automated inspection machine needs a concept of how the product-specific knowledge can be continuously incorporated into the optimization of the algorithms and made usable for the company over a long period of time.
About the Author
Felix Krumbein was responsible for the development of concepts for the qualification of inspection machines at Roche. At InspectifAI, he led the Visual Inspection division for the development of AI-based solutions for fully automated inspection machines. Mr. Krumbein is Head of the ECA Visual Inspection Group.