QPs in a Time of Electronic Data
The last few years have seen an acceleration in the transition of systems and processes into electronic formats. More and more companies have gone paperless and Cloud-based. Data streams have become data lakes. How can QPs take advantage of the overwhelming amount of data that's available to them, to improve their processes and efficiency?
During the QP forum in 2022, we took a quick poll from the audience to get an impression of the number of electronic systems that the delegates were using on a regular basis. The result was the figure on the right.
As can be seen, there is a large array of electronic systems in use in the pharmaceutical industry, and QPs make use of several of these in their daily work. Electronic quality management systems, enterprise resource planning systems, manufacturing execution systems, QC analytical reporting systems, data reporting systems, document management systems, etc… Practically all the work that a QP does, and systems that a QP uses, involve electronic systems and electronic data.
The "good-old days" of paper-based records and documentation are truly gone.
There are many advantages of this transition into electronic systems. The systems come with frameworks for the data, which ensure that the data are structured and used consistently. The data can be more easily filed, archived and retrieved, as compared to hardcopies. However, to be able to manage the lifecycle of the data effectively, from creation to archiving, retrieval, analysis and deletion or obsoletion, it is important to understand how the data are structured - the hierarchy of data - and where the data come from. Beginning from basic principles, the best place to start is the definition of the types of data that are used. The definitions used here are from the commonly accepted supply chain definitions. Slightly different definitions might apply in individual companies.
Transactional Data
- Describes business events, reflects daily operations - E.g. inventory movements, creating or changing documents or records
- Uniquely identifies each event (transaction) and is different for each event
- Once created, transaction data cannot be changed or edited
- Largest volume of data, used in reporting and data analysis
Transactional data can be entered manually into a system (e.g. results recorded by operator into a batch record) or automatically generated by a system (e.g. date and time stamp).
Transactional data can be transferred from one system to another, either manually (hardcopy to electronic system) or via an interface between electronic systems.
The method by which transactional data are created - automatically, interfaced or manually - will impact the risk of errors in the transactional data.
If the data are created automatically or interfaced, this should be validated appropriately to ensure that data are created or interfaced accurately and precisely.
If the data are manually entered or manually transcribed from one system to another, then a method of detection of errors would be advisable. Th is could be either automatic verification by the system against pre-set criteria, or a 2nd check by another person, which is recorded in the system.
An example of system check of manually entered data is where the system is pre-programmed with conditions on the data that are entered. E.g. manufacturing date cannot be a date in the future, date must be entered in particular format, etc.
Master Data
- Key business information supporting the transactions
- Describes the things the events are related to, e.g. customers, products, materials, suppliers, sites, etc
- Drives operational consistency
- Does not change over transactions
- Re-used for multiple purposes
- May be specific to operational systems, tailored to business processes
- Provides context to data reporting and analysis
Master data are defined by each company to suit their systems and processes. It is important to use the same consistent master data terms across systems within the same company, to avoid confusion (and enable sharing of data between systems).
This consistency and alignment of master data terms is particularly important when data are interfaced or transferred from one system to another system outside the company, as recently highlighted during the implementation of serialization - where companies had to upload data from their systems to the EMVS.
In recognition of the importance of consistency and alignment of master data terms, global initiatives are ongoing, with the objective of aligning and standardizing master data internationally. In the EU, the EMA is implementing the ISO standards for Identification of Medicinal Products (IDMP) in the EU master data roadmap and implementation of the Substance, Product, Organisations and Referential (SPOR) databases.
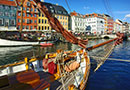
Recommendation
Copenhagen, Denmark28/29 May 2024
Quality Oversight
Table 1 shows some examples of Master and Transactional data, to show the differences between them.
Note that master data can also be a result of transactional data.
Business Process | Master Data | Transactional Data |
Manufacturing | Product/part number | Batch number |
Manufacturing | Shelf life (e.g. 24 months) | Expiry date (e.g. 31 May 2024) |
Document management | Document type: Procedure | Document number (e.g. SOP-123456 v 1.0) |
Quality records | Record type: Deviation | Deviation number (e.g. DEV-000010) |
Vendor management | Supplier | Vendor number (e.g. V000343) |
Order management | Customer | Customer number (e.g. 12345678) |
Inventory management | Warehouse location | Location number (e.g. L034-A-3) |
Inventory management | Stock unit | Number of units (e.g. 50000) |
Table 1: Examples of master and transactional data
For example, when a new customer is created in the order management system, the customer is assigned a Customer Number. The Customer Number is the Transactional data from the creation of the customer, and it is used to identify the customer for all subsequent transactions in the order management system. As the Customer Number is used for multiple purposes and remains unchanged through different transactions (e.g. sales orders), the Customer Number takes the characteristic of Master data in that it is used consistently across different transactions and does not change across different transactions. The same could apply to Supplier number.
Reference Data
- Subset of master data
- Referenced and shared by a number of systems
- Connects different domains and applications
- May be standardized to international or industrial standards
- Standardized within the company/enterprise
- Changes to reference data should be synchronized across the entire organization
These are the "special" master data elements that are used as reference points within a company, across companies and even throughout the industry.
Good examples are the master data elements that were standardized during the implementation of Serialisation, to enable transfer of data from organisations to EMVS data hubs.
Table 2 shows some examples of Master data that could also be Reference data.
Master Data element | Reference Data? | Explanation |
Product code | Yes | Product number is often standardized throughout the company (as part or SKU number) and sometimes also internationally (e.g. GTIN) |
Shelf life | Yes | Shelf life is standardized and used as a reference for multiple business processes |
Document type: Procedure | No | Document types and numbers are only used within the document management system of the company |
Record type: Deviation | No | Deviation numbers are only used within the deviation/quality management system of the company |
Supplier | Yes | Supplier number is referenced across multiple business processes |
Customer | Yes | Customer number is referenced across multiple business processes |
Warehouse location number | No | Warehouse location is only relevant for the individual warehouse |
Pack size | Yes | Pack size is often standardized throughout the enterprise and internationally (e.g. for serialization) |
Table 2: Examples of Reference data
Meta Data
- Data about the data
- Describes the who, what, where and when of data
- Maps the attributes of data
Examples of meta data are the data for audit trails, indexing search content, properties of files, etc.
Meta data is specific to each system that is used, and enable efficient sorting, collection, retrieval and organizing all the data that are generated or created for each system. Understanding how meta data are organized will be useful for trend analyses, data reporting, etc.
Knowledge of the meta data will enable us to find the data we're looking for, rather than having all the data dumped into a data lake and being lost in the depths.
Table 3 shows some examples of Meta data describing Transactional data.
Transactional Data | Meta Data |
Batch number | Audit trail – user, date, time stamp |
Expiry date | Audit trail – user, date, time stamp Possibly also change history |
Document number | Audit trail – user, date, time stamp Document properties – author, owner, approver, file size, type, file location, document version, etc |
Deviation number | Audit trail including change history Record properties – creator/initiator, size, workflow status, etc |
Vendor number | Audit trail including change history |
Customer number | Audit trail including change history |
Table 3: Examples of Meta data
Reporting Data
- Made of subsets of any master, reference, transactional or meta data
- Used for analyzing operational and company process performance
- Determined by the company based on the performance indicators to be measured or decisions to be made
This is the set of data elements that have been identified as "markers" to enable the related raw data to be pulled and presented in various formats for data reporting and analysis.
Reporting data sets can be selected based on the reports to be generated. These sets can be changed according to business needs, in alignment with the Key Performance Indicators (KPIs) that are chosen by the company.
Why is this important to QPs?
As QPs are using more and more electronic systems, there is a risk of being overwhelmed by the masses of data that are generated. If QPs understand the hierarchy of data and how the data are generated, they will be able to identify which data can be trusted, which data should be verified to assure that systems are working properly.
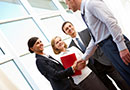
Recommendation
Munich, Germany4-6 June 2024
Qualified Person Education Course Module A PLUS IMP Pre-Course Session
This knowledge of the data hierarchy will help QPs (and QA managers) focus their efforts and attention on the right data elements and processes.
For example, in setting up master data such as shelf life of products or materials, the emphasis could be placed on ensuring that the correct shelf life is entered in the master data, so that all subsequent expiry date calculations done by the system can be trusted implicitly.
Equally, if transactional data are not generated automatically by a validated system or interfaced via a validated software interface, but are entered or transcribed manually, then more attention might be necessary to ensure accuracy and completeness of the manual data entry or transcription.
With the increased understanding of the way data are structured, QPs could better interrogate the KPI results that are reported by looking critically at the reporting data sets, to assure themselves that the processes and quality management systems of the company are performing as well as expected, hence aiding them in fulfilling their responsibilities as QP.
About the Author
Cheryl Chia is a Consultant for GMP and GDP compliance in the pharmaceutical supply chain at Lotus Phoenix Consulting, Netherlands and member of the EQPA Board of Directors.